A hierarchical machine learning approach for point cloud segmentation
Keywords:
point cloud, classification, hierarchical segmentation, machine learningAbstract
The recent years saw an extensive use of 3D point cloud data for heritage documentation, valorisation, and visualisation. Although rich in metric quality, these 3D data lack structured information such as semantics and hierarchy between parts. In this context, the introduction of point cloud classification methods can play an essential role for better data usage, model definition, analysis, and conservation. The paper aims to extend a machine learning (ML) classification method with a multi-level and multi-resolution (MLMR) approach. The proposed MLMR approach improves the learning process and optimises 3D classification results through a hierarchical concept. The MLMR procedure is tested and evaluated on two large-scale and complex datasets: the Pomposa Abbey (Italy) and the Milan Cathedral (Italy). Classification results show the reliability and replicability of the developed method, allowing the identification of the necessary architectural classes at each geometric resolution.
Downloads
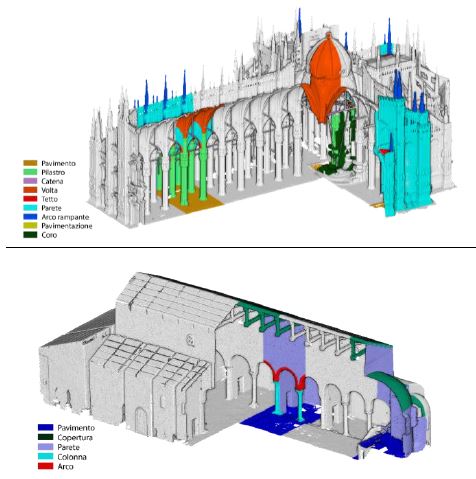
Downloads
Published
How to Cite
Issue
Section
License
Copyright (c) 2020 Eleonora Grilli

This work is licensed under a Creative Commons Attribution-ShareAlike 4.0 International License.