Learning from synthetic point cloud data for historical buildings semantic segmentation
Abstract
In the historical heritage scenario, semantic segmentation of 3D point clouds is gaining more and more attention, since it might help to automatically recognize historical architectural elements. However, semantic segmentation is particularly challenging in historical and classical architecture, due to the shapes complexity and the limited repeatability of elements across different buildings, which makes it difficult to define common patterns within the same class. Furthermore, as deep learning models requires a considerably large amount of annotated data to be trained and tuned in order to properly handle unseen scenes, the lack of (big) publicly available annotated point clouds in the historical building domain is a huge problem, blocking the research in this direction. On the other hand, creating a critical mass of annotated point clouds by manual annotation is very time-consuming and impractical.
For this purpose, in this work we explore the idea of leveraging synthetic point cloud data to train deep learning models to perform semantic segmentation of point clouds obtained via Terrestrial Laser Scanning (TLS). To achieve this purpose, we present an improved version of the Dynamic Graph CNN (DGCNN) named RadDGCNN. In our experiments, we evaluate the trained models on synthetic dataset (publicly available) about two different historical buildings: the Ducal Palace in Urbino, Italy, and Palazzo Ferretti in Ancona, Italy. RadDGCNN yields good results, demonstrating improved segmentation performances on the TLS real datasets.
Downloads
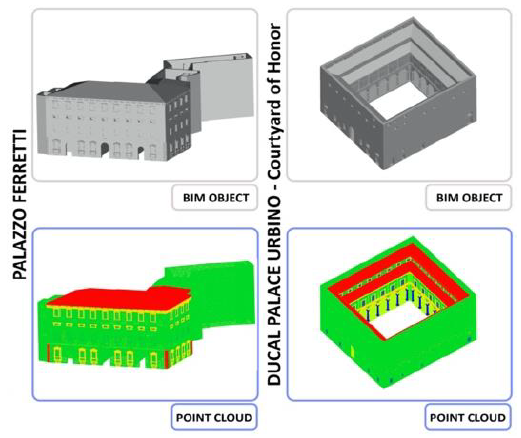
Downloads
Published
How to Cite
Issue
Section
License
Copyright (c) 2020 Roberto Pierdicca

This work is licensed under a Creative Commons Attribution-ShareAlike 4.0 International License.